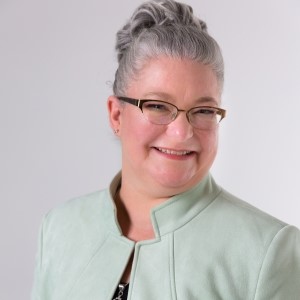
Karen O’Brien
Senior Principal Data Scientist
Modern Technology Solutions, Inc
Karen O’Brien is a senior principal data scientist and Artificial Intelligence and Machine Learning (AI/ML) practice lead at Modern Technology Solutions, Inc. In this capacity, she leverages her 20-year Army civilian career as a scientist, tester and evaluator, Operations Research/Systems Analyst (ORSA), and analytics leader to aid Department of Defense (DoD) agencies in implementing AI/ML and advanced analytics solutions. Her Army analytics career ranged ‘from ballistics to logistics,’ with a preponderance of time supporting the U.S. Army Test and Evaluation Command where she was known for designing scientific test and evaluation for emerging technologies. She was a physics and chemistry nerd in the early days, but now uses her M.S. in Predictive Analytics from Northwestern University to help her DoD clients tackle the toughest analytics in support of national defense. She is co-lead of the Women in Data Huntsville Chapter, a guest lecturer in data and analytics graduate programs, and an ad hoc study committee member at the National Academy of Sciences.