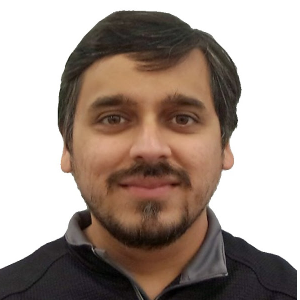
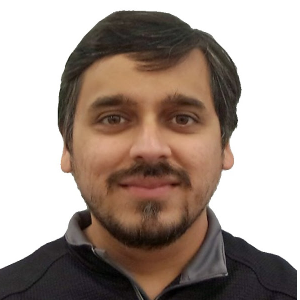
For product companies, it is important to be able to forecast product return rates to perform provisioning without having to maintain a surplus inventory. A common way to predict return rates is through modeling product returns using survival models, which operationally amounts to the fitment of a Weibull distribution to the product returns until a certain cut-off date.For all hardware and devices companies Weibull holds gold standard for forecasting the return rate . In this presentation , We aim to deep dive on consumer returns , highlight nuances where weibull fails to provide accurate forecast and show how we feature engineer those cases in order to produce accurate forecasts