Plenary Speakers
Sunday, July 20
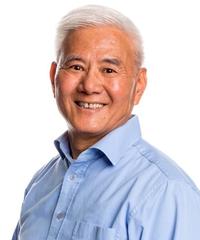
Yinyu Ye
Mathematical Optimization in the Era of AI
This talk aims to present several mathematical optimization problems/algorithms for AI such as the LLM training, tunning and inferencing. In particular, we describe how classic optimization models/theories can be applied to accelerate and improve the Training/Tuning/Inferencing algorithms that are popularly used in LLMs. On the other hand, we show breakthroughs in classical Optimization (LP and SDP) Solvers aided by AI-related techniques such as first-order and ADMM methods, the low-rank SDP theories, and their implementations on GPU.
Monday, July 21
Sheldon H. Jacobson
AI Up in the Sky: The Future of Aviation Security
The United States Transportation Security Administration (TSA) secures over 400 commercial airports around the nation to keep the air system secure from threats. They have historically been threat detection centric, working to keep threat items off airplanes. Since the launch of TSA PreCheck program in 2011, a risk-based system that matches security resources with passenger risk, their focus has shifted, working to separate the large pool of benign passengers from the handful of bad actors. This talk provides an overview of airport security in the United States and how biometrics like facial recognition fits into the risk-reduction calculus for airport security. A roadmap for the future of airport security is provided that relies on facial recognition that will transform airport security from a process of stopping prohibited items from entering the air system to reducing air system risk by better knowing the population of air travelers and identifying risky passengers prior to them entering the air system.
Tuesday, July 22
Ngien Hoon Ping
Transforming Mobility:
SMRT’s Journey in Data and Digital Innovation
In today’s fast-evolving landscape, digital transformation is not just an option – it is a strategic imperative. SMRT is driving digital transformation by leveraging analytics, artificial intelligence, management science and digital technologies to address complex operational and business challenges.
Drawing on practical examples from SMRT and beyond, this session will showcase how data-driven decision-making, predictive analytics and digital solutions are improving operations effectiveness, safety, and customer-centricity in the fields of public transportation, mobility, and supply chains. Building a future-ready organization starts with putting data and digital at the heart of the business.
Keynote Speakers
Monday, July 21
Teo Chung Piaw
From Theory to Impact: Building Data-Driven Models for Real-World Optimization
This talk highlights recent efforts to build theory- enabled, data-driven models for optimizing complex operational decisions across multiple sectors, with a focus on bridging the gap between rigorous analytical frameworks and real-world implementation. A core emphasis of our work is on developing models that not only leverage data effectively but also provide robust performance in volatile environments where uncertainty and rapid change are inherent. A central methodological advancement lies in the theory of moments, which we extend to develop tractable distributionally robust optimization frameworks. These models allow decision-makers to account for ambiguity in demand, supply, or pricing dynamics by using limited statistical information (e.g., means, variances) without relying on precise distributional assumptions. This is particularly valuable in environments with high volatility, where classical optimization approaches may fail to perform reliably. We apply this advancement in multiple domains, such as airline revenue management, where it informs real-time pricing and offer decisions under uncertain demand, and in logistics and humanitarian operations, where we model resource allocation and routing under unpredictable disruptions. This work reflects the growing importance of operations research (OR) in Singapore’s innovation ecosystem, where data and theory converge to solve practical challenges. It also demonstrates how theory-to-practice translation can create scalable, high-impact decision tools for sectors ranging from aviation to public services, especially in an era defined by volatility and complexity.
Jonathan Chang
Semiconductor Manufacturing Development – past, present and future
Semiconductor manufacturing is a complicated integrated process where even a single step—such as wafer testing involving man, machine, material, and method (4M)—can trigger multiple deviations, impacting key performance metrics like quality, speed, productivity, and cost. A completed wafer fabrication involves hundreds to thousands of steps will produce hundreds of deviations for overall process. Beyond these factors, the growing complexity of advanced process technologies (from 0.35µm to 5nm) has exponentially increased challenges in yield management, driven by interactions between device layers (e.g., OD–Poly–Metal) and production modules (lithography, etch, thin-film, diffusion, and CMP). A high-volume manufacturing facility must simultaneously manage hundreds of thousands of wafers, including production, R&D, and quality assurance lots—while maintaining competitive cycle times (CT) for customers and ensuring optimal machine productivity to control costs. Over past 30 years, the semiconductor foundry industry has demonstrated that effective deviation management is the core of manufacturing capability. This capability ultimately differentiates companies that sustain long-term competitiveness, enabling them to evolve into leading semiconductor technology providers and secure market leadership. This presentation will explore the evolution of semiconductor manufacturing capability, highlighting how industrial engineering (IE) and information technology (IT) establish the foundational capabilities required for world-class semiconductor production.
Kelvin Bryan Tan
Operations Research for Pandemic Preparedness: Lessons from COVID
COVID was unprecedented in it’s global impact of human lives and impact on healthcare strain, as well as the economic disruption as a result of mitigation measures. Operations Research played an important role in many of the critical aspects in the management of the pandemic by informing resource allocation, logistics and distribution, supply chain management, optimization of testing strategies and decision analysis. How successful were we and what has been the contribution of Operations Research? More importantly, how can we do better for the next pandemic?
Tuesday, July 22
Stefan Wörner
Quantum Computing and Operations Research: Potential, Challenges, and the Path Forward
Quantum computing is a new computational paradigm with the potential to transform many disciplines, with optimization often being mentioned as a very promising candidate. In this talk, I will discuss the state of quantum computing today and explore both its potential and challenges for optimization. I will highlight opportunities for quantum advantage, as well as how classical optimization can support progress in quantum computing. A critical step towards achieving quantum advantage is identifying the right problems to target. To this extent, I will discuss efforts in systematic benchmarking and outline new research directions for advancing quantum optimization.
Ming Hu
Supply-Demand Matching in Space
This talk provides an overview of recent efforts in building models and theories for supply and demand matching over a space. First, we will model recent applications with spatial movements in the on-demand economy and global supply chains to extend the scope of urban operations research/supply chain management and draw managerial insights on decisions such as pricing and matching in managing those systems. Second, we will develop theories on spatial resource allocation and general choice modeling by drawing connections with the classic optimal transport (OT) problem.
Sharon Ng
Bridging the Divide: Advancing Knowledge Through Industry-Academia Collaboration
In an era marked by rapid technological transformation and increasingly complex societal challenges, the need for robust collaboration between academia and industry has never been more critical. I will address the enduring tension and transformative potential of bridging the academic-industry divide. While scientific rigor forms the bedrock of credible knowledge production, relevance to real-world application ensures that such knowledge translates into meaningful societal and economic impact. Though achieving both simultaneously is inherently challenging, neither domain can thrive in isolation. Progress demands deliberate alignment and sustained partnership. I would like to advocate for deeper, more structured collaborations that not only enrich scholarship but also enhance innovation and inform practice. Only by co-creating knowledge that is both rigorous and relevant can we address the pressing needs of our time and shape a more sustainable and inclusive future.