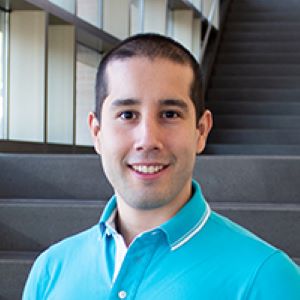
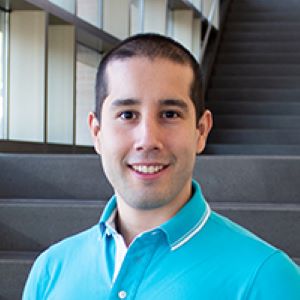
Warehouse dock and trailer loading operations carry safety risks for employees, such as improperly secured loads that may cause accidents during handling. Further, non-compliance to safety protocols can also lead to significant throughput reduction. Ensuring adherence to Standard Operating Procedures (SOPs) is challenging, especially without feedback mechanisms. Measuring individual performance or identifying safety violations is often difficult, as existing techniques can be intrusive, interfere with the human-machine interface, or rely heavily on subjective evaluation.We present novel computer vision (CV) techniques to identify trailer loading strap settings during loading operations, comparing them against SOPs. We validate compliance, establish a feedback loop, and prevent truck departures with non-compliant loads.With an 85% success rate in detecting non-compliant trailers and a 1.7% false detection rate, the model is robust against poor-quality images and determinines the minimum dataset size for reliable results. Deployed at 1,900+ Amazon sites, the CV model enhances safety and operational efficiency.