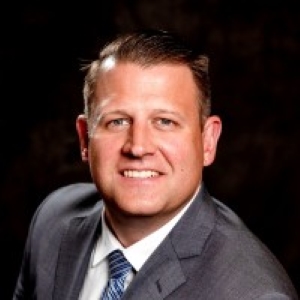
Kevin Larrabee
Head of Product
DataShapes AI
Kevin Larrabee joins DataShapes AI as the Head of Product. He recently transitioned as the Chief Data Officer of a U.S. Army Special Operations Command (USASOC) Research and Development organization. He led the Command’s technical partnerships, enabled capability development, and set strategy towards making data an enterprise asset.
Kevin combined his leadership abilities, strategic vision, and technical prowess throughout his career to solve the nation’s most complex problems. He has innovated across the Army Staff, CENTCOM, and USASOC. He was the first data scientist to deploy in support of the Afghanistan National Army Special Operations Command in 2015, create a CENTCOM wargaming and human dynamic modeling team in 2017, and build a multi-functional data team in 2020.
Under Kevin’s dynamic leadership at USASOC, the data directorate has experienced a remarkable transformation. His entrepreneurial spirit has propelled the team’s growth from a modest 3 to a robust 30 personnel while catapulting the data budget from $500K to an impressive $10M. He has overseen eight concurrent machine learning and software development projects, facilitating advanced technology’s rapid adoption, building, and integration.
Kevin holds a B.S. in Business Management from the U.S. Military Academy, an M.S. in Operations Research from the U.S. Naval Postgraduate School, and an MBA from Winston-Salem State University. He travels, invests, and coaches his children’s sports teams in his free time.