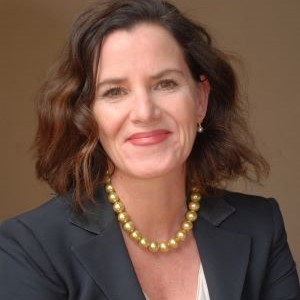
Paula Payton
Senior Manager Center for Machine and Data Science
Deloitte Services LLP
Paula is a Senior Manager with Deloitte Services LLP where she leads the Center for Machine Intelligence and Data Science (MInDS), a multidisciplinary team of data scientists ,behavioral scientists, and survey specialists that support thought leadership from seven major research centers at the firm
A technical leader, management expert, social scientist, and educator, she has held leadership roles in both industry and academia and is expert at using data, analytics and behavioral science to help organizations unlock insights .
In addition to her industry work, she is a longstanding data acience and analytics professor and active in teaching and guiding the next generation of analytics , data science and AI talent. She has held academic appointments at Wake Forest University School of Business, Columbia University and NYU, and mentors graduate students at the University of Chicago ‘s Data Science Institute. and Columbia Data Science Society.
Her current research interests include developing AI tools to transform research and analysis, as well as incorporating behavioral science insights into AI models and systems
Paula holds a PhD in Management (NEOMA Business School), and an MA in Behavioral Science (University of Chicago) with graduate diplomas in data science (Johns Hopkins University) and corporate strategy (Cornell University University Johnson School of Management)