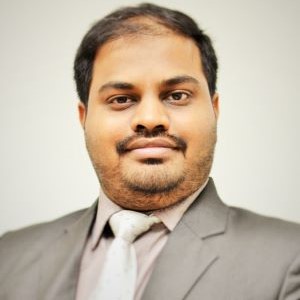
Sandeep Vellanki
Manager, Data Science Team
Deloitte Services LLP
Sandeep Vellanki is a Data Science manager leading a team focused on transforming complex data into actionable insights. With over 13 years of experience, Sandeep specializes in machine learning, predictive modeling, and data visualization, delivering impactful solutions across industries. He excels at bridging the gap between technical and non-technical stakeholders, translating intricate data concepts into clear, actionable insights.
Sandeep has been at the forefront of emerging technologies, particularly in Natural Language Processing (NLP) and generative AI. Notable projects include developing several generative AI-powered tools at Deloitte which customizes content and enhances client-facing teams’ efficiency. His work on the QRAG architecture has also improved the performance of retrieval-augmented generation (RAG) models by enhancing context pulling and specificity.
Sandeep holds an MBA in Marketing from IMT-Ghaziabad and an undergraduate degree in Engineering from VNR VJIET, JNTU, Hyderabad.