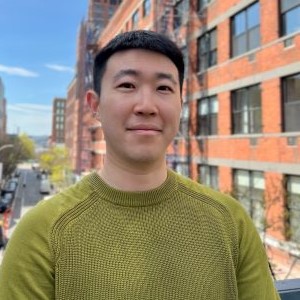
Sitao Zhang
Lead Data Scientist, AI
Johnson & Johnson
Sitao Zhang is a Data Science Lead at Johnson & Johnson, where he has spent nearly eight years driving innovation in the healthcare supply chain. His work focuses on applying cutting-edge data science techniques—including natural language processing, traditional machine learning, deep learning, and mathematical optimization—to solve real-world challenges and advance Johnson & Johnson’s Credo-driven mission. Sitao is passionate about bridging advanced technologies with practical impact in cross functional domains. Outside of work, he enjoys staying active with family through running and CrossFit