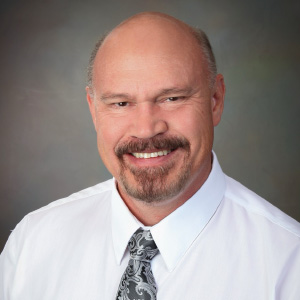
Thor Osborn, CAP
Principal Systems Research Analyst
Sandia National Laboratories
He focuses his data science efforts on analysis and operations research to inform executive leadership on key business decisions. Most of his recent work has been in workforce behavioral studies, modeling and analysis, compensation, and document management. He holds a doctorate in biomedical engineering from the University of Washington and an MBA from the University of New Mexico, and is a Certified Analytics Professional. He has been awarded three US patents and has published on diverse topics including biological sensing, MEMS actuators, seeker systems, analysis of terrorism, and workforce analysis.