Tutorials are designed to disseminate timely work by leading researchers and are highly valued by the attendees. They often include an in-depth, state-of-the-art lay-of-the-land of a field, given by an expert. Tutorials are a great resource for newcomers to a field but they also provide a comprehensive review for others who are familiar with the topic. This year, we have planned multiple tutorials on a broad spectrum of topics, ranging from fire management and inverse optimization to random graphs and artificial intelligence. We look forward to seeing several of you at these tutorials.
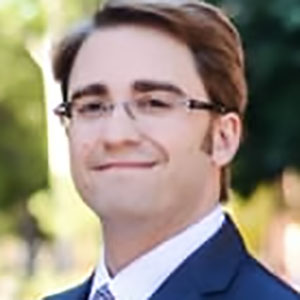
The Continuous Approximation Paradigm in Logistics Systems Analysis
John Carlsson, University of Southern California
The continuous approximation (CA) paradigm has been an effective tool for obtaining managerial insights in logistics problems since the seminal papers of Few and Beardwood, Halton, and Hammersley in the 1950s. The core concept in CA is that one replaces detailed data in a problem instance with concise algebraic expressions. This tutorial will provide an overview of recent advancements in this area, as well as promising future research directions.
Inverse Optimization: Theory and Application
Timothy Chan, University of Toronto
Inverse optimization describes a process that is the “reverse” of traditional mathematical optimization. Unlike traditional optimization, which seeks to compute optimal decisions given an objective and constraints, inverse optimization takes decisions as input and determines an objective and/or constraints that render these decisions approximately or exactly optimal. In recent years, there has been an explosion of interest in the mathematics and applications of inverse optimization. This tutorial will provide a comprehensive introduction to the theory and application of inverse optimization.
O.R. and Analytics for Public Policy: Lessons from the Pandemic
Peter Frazier, Cornell University
The COVID-19 pandemic laid bare gaps in governments’ ability to base policy on data and quantitative reasoning. This talk argues that O.R. and analytics can help craft more effective policy and that the COVID-19 crisis has created a window of opportunity for change. We draw lessons from the speaker’s experience at Cornell University, where OR is a fundamental part of the university’s pandemic response. We focus on practical tools and techniques for policy-focused O.R.-based decision support in public health and other public- and private-sector decision domains.
Wildfire Management: An Operational Research Perspective
David Martell, University of Toronto
Fire is a natural component of many forest ecosystems but wildfires can have very significant detrimental impacts on people, property, forest resources and infrastructure. British Columbia’s 2021 fire season is of course, a recent poignant example. Fire cannot nor should it be eliminated from the forest but that poses complex challenges to those that live with fire and those that manage fire. I will provide a brief overview of wildfire management, describe some fire management problems I have studied, and identify some important open problems I believe operational researchers can help resolve.
Opinion Dynamics on Directed Random Graphs
Mariana Olvera-Cravioto, University of North Carolina
A popular way of modeling the exchange of information among individuals in a society is to use a large random graph whose vertices represent the individuals and whose edges represent acquaintances or friendships. Once the graph is realized we can model the exchange of information by defining a Markov chain on the graph whose transition probabilities determine how individuals will update their opinions once they listen to those of their acquaintances. If the listening relationship between individuals is not symmetric, we can assume the graph is directed. This tutorial will explain how to model and analyze opinion dynamics using the DeGroot-Friedkin model on directed random graphs, with the goal of proving conditions under which either consensus or polarization occurs. The techniques presented in the tutorial also extend to the analysis of a wide class of Markov chains on directed random graphs.
Markov Decision Processes in Health Care
Steven Shechter, University of British Columbia
Markov Decision Processes (MDPs) provide a rich framework for sequential decision making under uncertainty. This tutorial will introduce theory, algorithms, and health care applications of MDPs. It will begin with basic MDP concepts, solution methods, and structural properties, and then provide overviews of two major extensions of MDPs: Partially Observable MDPs, and Reinforcement Learning. Applications in health care will be interspersed throughout, covering both system-level (i.e., resource allocation) and patient-level (i.e., medical decision making) models.
Analytics for Social Impact
Phebe Vayanos, University of Southern California
We discuss work in collaboration with community partners and policymakers focused on homelessness and public health in vulnerable communities. We present research advances to address one key cross-cutting question: how to assign scarce intervention resources while accounting for the challenges of open world deployment? We show concrete improvements over the state of the art based on real world data. We are convinced that by pushing this line of research, analytics can play a crucial role to help fight injustice and solve complex problems facing our society.
Causal Inference in the Presence of Network Interference
Christina Lee Yu, Cornell University
Randomized experiments are widely used to estimate causal effects of proposed “treatments” in domains spanning the physical and biological sciences, social sciences, engineering, medicine and health, as well as in public service domains and the technology industry. However, classical approaches to experimental design rely on critical independence assumptions that are violated when the outcome of an individual A may be affected by the treatment of another individual B, referred to as network interference. This interference introduces computational and statistical challenges to causal inference. In this tutorial, we will survey the challenges of causal inference under network interference and the different approaches proposed in the literature to account for network interference. We will present a new hierarchy of models and estimators that enable statistically efficient and computationally simple solutions under nonparametric polynomial models, with theoretical guarantees even in settings where the network is completely unknown, the data is observational, or the model is misspecified.