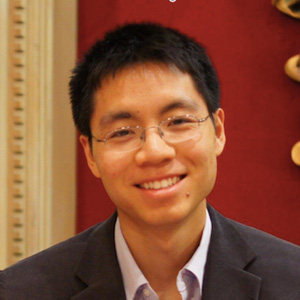
Louis Chen
Louis Chen
Asst. Professor, Operations Research at Naval Postgraduate School
Track: Machine Learning & AI
On the Adversarial Robustness of Benjamini-Hochberg
The Benjamini-Hochberg (BH) procedure is widely used to control the false detection rate (FDR) in large-scale hypothesis testing. We investigate the effect that adversarial perturbations may have on this control; in particular, we demonstrate that the FDR guarantee can be badly broken with minimal perturbation to a single p-value, highlighting the fragility of the BH procedure. We focus on identifying the perturbation of a single p-value that generates the largest adversarially-adjusted FDR, and provide: (1) an efficient (linear-time) optimal algorithm; (2) non-asymptotic guarantees on the expected adversarial-adjustment to FDR; and (3) numerical experiments on data sets to illustrate this effect. The takeaway is that the probabilistic dependence among the reject/accept decisions generated by the BH procedure can be hacked. Our technical analysis involves reframing the BH procedure as a “balls into bins” process, and drawing a connection to generalized ballot problems that facilitates an information-theoretic approach for deriving non-asymptotic lower bounds.