Plenaries
Sunday
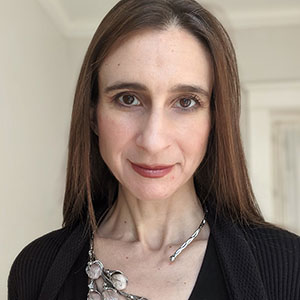
Cynthia Rudin
Do Simpler Machine Learning Models Exist and How Can We Find Them?
While the trend in machine learning has tended towards building more complicated (black box) models, such models are not as useful for high stakes decisions – black box models have led to mistakes in bail and parole decisions in criminal justice, flawed models in healthcare, and inexplicable loan decisions in finance. Simpler, interpretable models would be better. Thus, we consider questions that diametrically oppose the trend in the field: for which types of datasets would we expect to get simpler models at the same level of accuracy as black box models? If such simpler-yet-accurate models exist, how can we use optimization to find these simpler models? In this talk, I present an easy calculation to check for the possibility of a simpler (yet accurate) model before computing one. This calculation indicates that simpler-but-accurate models do exist in practice more often than you might think. Also, some types of these simple models are (surprisingly) small enough that they can be memorized or printed on an index card.
About Cynthia Rudin
Cynthia Rudin is a professor of computer science, electrical and computer engineering, statistical science, mathematics, and biostatistics & bioinformatics at Duke University. She directs the Interpretable Machine Learning Lab, whose goal is to design predictive models with reasoning processes that are understandable to humans. Her lab applies machine learning in many areas, such as healthcare, criminal justice, and energy reliability. She holds an undergraduate degree from the University at Buffalo, and a Ph.D. from Princeton University. She is the recipient of the 2022 Squirrel AI Award for Artificial Intelligence for the Benefit of Humanity from the Association for the Advancement of Artificial Intelligence (the “Nobel Prize of AI”). She is a fellow of the American Statistical Association, the Institute of Mathematical Statistics, and the Association for the Advancement of Artificial Intelligence. Her work has been featured in many news outlets including the NY Times, Washington Post, Wall Street Journal, and Boston Globe.
Monday
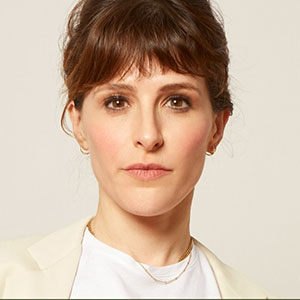
Maxine Bédat
The Life and Death of Your Jeans
Maxine Bédat, founder and Director of think and do tank, New Standard Institute and author of Unraveled: The Life and Death of a Garment, an FT Business Book of the Year, will share the hidden world behind our clothing, highlighting key problems within our long supply chains, research that needs to be undertaken, and systems solutions that management science can champion.
About Maxine Bédat
Invited by the INFORMS Diversity, Equity, and Inclusion Committee, Maxine Bédat is the founder and director of The New Standard Institute. Prior to NSI, Maxine co-founded and was the CEO of the fashion company Zady. For its work in sustainability, Zady was named one of the world’s “Most Innovative Companies” in retail by Fast Company. Bédat has been recognized by Oprah in her Super Soul 100, for leaders elevating humanity, serves as an ambassador for Rainforest Alliance and is on the Council of NationSwell. She has spoken at some of the world’s leading conferences, including the WWD Apparel/Retail CEO Summit, and has been regularly featured as an expert by Bloomberg, Forbes, Business of Fashion, CNN and the Huffington Post. Bédat began her career in international law working at the Rwandan Criminal Tribunal and received a Juris Doctor from Columbia Law School.
Tuesday
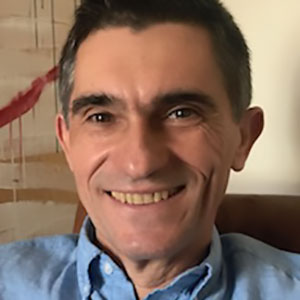
Patrick Jaillet
Online Optimization and Learning for Sequential Decision-Making
There are many situations in which present actions must be made and resources allocated with incomplete knowledge of the future. It is not clear in this setting how to measure the quality of a proposed decision strategy. Online optimization compares the performance of a strategy that operates with no knowledge of the future (on-line) with the performance of an optimal strategy that has complete knowledge of the future (off-line). In some cases, probabilistic information about the future may be available or learned. In this talk, we provide an overview of some results obtained from that perspective on various classical sequential decision-making problems.
About Patrick Jaillet
Patrick Jaillet is the Dugald C. Jackson Professor in the Department of Electrical Engineering and Computer Science and a member of the Laboratory for Information and Decision Systems at MIT. He is also co-Director of the MIT Operations Research Center and holds a joint appointment in the Operation Research and Statistics Group at MIT Sloan. Dr. Jaillet’s research interests include online optimization and learning; machine learning; and decision making under uncertainty. Dr. Jaillet is the recipient of many research and teaching awards. He is a Fellow of the Institute for Operations Research and Management Science Society (INFORMS), a member of the Mathematical Optimization Society (MOS), and a member of the Society for Industrial and Applied Mathematics (SIAM).
Wednesday
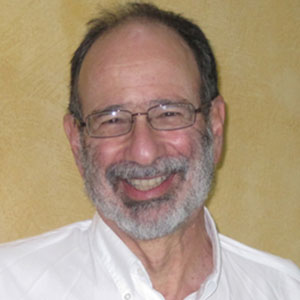
Alvin E. Roth
Market Design: The Dialog Between Simple Abstract Models and Practical Implementation
I’ll review some of the elegantly simple models that underlie the initial designs for matching processes like the medical residency Match, school choice and kidney exchange, and the modifications, complications and computations that were needed to get new designs adopted, implemented and maintained over the years.
About Alvin E. Roth
Al Roth is the Craig and Susan McCaw Professor of Economics at Stanford University, and the George Gund Professor Emeritus of Economics and Business Administration at Harvard. His research is in game theory, experimental economics, and market design. Among the markets he has designed (or, in this case, redesigned) is the National Resident Matching Program, through which most doctors find their first employment as residents at American hospitals. He has also helped in the reorganization of the market for more senior physicians, as they pursue subspecialty training, and in other labor markets. He helped design the high school matching system used in New York City, and the school matching systems used in Boston, Denver, and New Orleans. He is one of the founders and designers of kidney exchange in the United States, which helps incompatible patient-donor pairs find life-saving compatible kidneys for transplantation. He shared the 2012 Nobel memorial prize in Economics. He was also awarded the 2021 Philip McCord Morse Lectureship Award.
Keynotes
Sunday
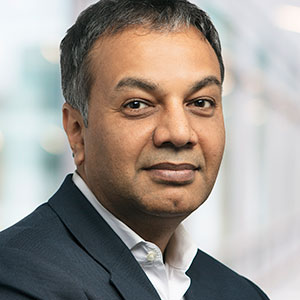
Prashant Yadav
Global Health Security and Healthcare Supply Chains: Perspectives and Opportunities for Operations Research
As has become abundantly obvious in the last two years, infectious diseases have the potential to endanger lives, disrupt economies, travel, trade, and have a significant impact on our mental health. The need for robust prevention, preparedness, and response mechanisms is also widely recognized and accepted. However, achieving global health security also requires coordinated actions across countries, regions, and other forms of administrative geographical units. Operations research plays a key role in many parts of global health security such as modelling and simulation of disease outbreaks, analytical models of different policy responses, and design and operation of supply chains for medical counter measures. While all aspects of global health security depend on global coordination, the supply chains for global health technologies such as vaccines, diagnostics, and therapeutics require special kinds of coordination across multiple manufacturers, purchasers, payers, and delivery partners. This talk will focus on the evolution of the field of global health supply chains, which had it origins in global health security, but over time focused more on health systems building. It will highlight areas where health care supply chain research has contributed at the forefronts of policy making regarding global pandemic response and preparedness. It will also present opportunities for OR/OM researchers to contribute more effectively to achieve direct policy impact in this area.
About Prashant Yadav
Prashant Yadav is a globally recognized scholar in the area of healthcare supply chains. He is an Affiliate Professor at INSEAD, Senior Fellow at the Center for Global Development, and Lecturer at Harvard Medical School. He is the author of many peer-reviewed scientific publications and his work has also been featured in prominent print and broadcast media such as the BBC, New York Times, CNN, Financial Times, WSJ and NPR. In addition to his roles in academia and think-tank, Prashant serves on the boards of many health and development focused companies. In his previous roles Prashant has worked as Strategy Leader-Supply Chain at the Bill & Melinda Gates Foundation; Vice President of Healthcare at the William Davidson Institute and Faculty at the Ross School of Business at the University of Michigan; Professor of Supply Chain Management at the MIT-Zaragoza International Logistics Program and Research Affiliate at the MIT Center for Transportation and Logistics. Yadav has been asked for expert testimony on medicine supply chains in the US Congress and Parliaments/Legislative bodies of many countries He works closely with and advises many country governments, and philanthropic organizations on healthcare supply chain strategy. Yadav trained as a Chemical Engineer and obtained his PhD in Management Science & Operations Research.
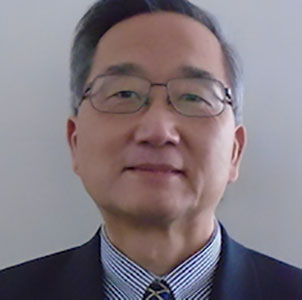
David Yao
Modeling Systemic Risk in Supply-Demand Networks
Recent events (the pandemic, geo-political conflicts, climate change, etc) call for studies on systemic risk in supply-demand networks (SDNs). An SDN is a network with nodes (or “agents”) representing resources with processing and/or storage capabilities and arcs representing their supply-demand relations. Systemic risks in the SDN arise from its interconnectedness, such that disruption (or “shock’’) at one node may quickly propagate to other nodes and possibly lead to a system-wide disaster. There are similarities to systemic risk in the financial system, but also fundamental differences. We will discuss how stochastic networks can play an essential role in modeling and analyzing systemic risk in the SDN, along with certain risk-hedging tools and other technologies such as digital twins and reinforcement learning.
About David Yao
David D. Yao is the Piyasombatkul Family Professor of Industrial Engineering and Operations Research at Columbia University, where he is a co-chair of the Financial and Business Analytics Center at Columbia Data Science Institute, and more recently, co-director of the Columbia Fintech, AI and Business Analytics (FABULYS) Initiative. His research and teaching interests are in applied probability and stochastic networks, focusing on resource control and risk analysis. He has been a Guggenheim Fellow, IEEE Fellow, INFORMS Fellow, and a Member of the National Academy of Engineering.
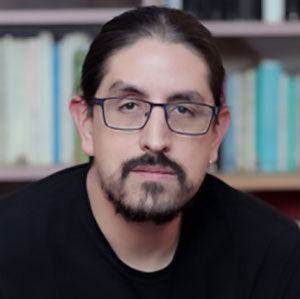
Leonardo J. Basso
2022 Edelman Reprise: Analytics Saves Lives during the Covid Crisis in Chile
During the Covid-19 crisis, the Chilean Ministry of Health and the Ministry of Sciences, Technology, Knowledge and Innovation partnered with the Instituto Sistemas Complejos de Ingenier´ıa (ISCI) and the telecommunications company ENTEL, to develop innovative methodologies and tools that placed operations research and analytics at the forefront of the battle against the pandemic. These innovations have been used in key decision aspects that helped shape a comprehensive strategy against the virus, including tools that: (i) shed light on the actual effects of lockdowns in different municipalities and over time; (ii) helped allocate limited intensive care capacity; (iii) significantly increased the testing capacity and provided on-the ground strategies for active screening of asymptomatic cases; and (iv) implemented a nationwide serology surveillance program that significantly influenced Chile’s decision regarding vaccine booster doses and that also provided information of global relevance. Important challenges during the execution of the project included the coordination of large teams of engineers, data scientists, and health care professionals in the field; how to effectively communicate information to the population; and the handling and use of sensitive data. The initiatives enjoyed ample press coverage and, by providing scientific evidence supporting the decision-making behind the Chilean strategy against the pandemic, they helped provide transparency and objectivity to decision-makers and the general population. According to conservative estimates, the number of lives saved by all of the initiatives together is close to 3,000, equivalent to more than 5% of the total death toll in Chile during the pandemic. The saved resources associated with testing, ICU beds, and working days amount to more than 300 million USD.
Chile Team Members
Leonardo J. Basso, Instituto Sistemas Complejos de Ingeniería (ISCI) & Universidad de Chile (Primary Speaker)
Marcelo Olivares, Instituto Sistemas Complejos de Ingeniería (ISCI) & Universidad de Chile
Denis Sauré, Instituto Sistemas Complejos de Ingeniería (ISCI) & Universidad de Chile
Charles Thraves, Instituto Sistemas Complejos de Ingeniería (ISCI) & Universidad de Chile
Gabriel Weintraub, Stanford University
About Leonardo J. Basso
Leonardo J. Basso is Full Professor at the Department of Civil Engineering, and the Director of the ANID Center of Excellence Instituto Sistemas Complejos de Ingeniería (ISCI), a multi university center devoted to research, innovation and societal impact. His research areas broad, ranging from transportation and urban economics, industrial organization and competition policy, to analytics applied to health issues, particularly COVID.
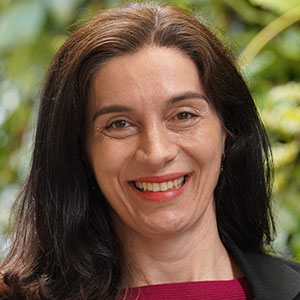
Luciana Buriol
IFORS Distinguished Lecturer
The Amazon Fulfillment Network: Topology and Capacity Planning
The Amazon fulfillment network is comprised of Amazon buildings and transportation lanes among them. In this talk, I will focus on the network with nodes representing Amazon Fulfillment Centers, Sortation Centers, and the Delivery Stations, where the links are the truck and air lanes among them. The lanes of this network are highly dynamic: their capacity can be changed a few days in advance, and they can also be deprecated or launched with short notice. On the other hand, large buildings are planned years ahead. Designing and expanding this network, as well as adjusting the nodes and links capacities for everyday operation demands an orchestration of complex OR systems. In this talk, I will describe this process.
About Luciana Buriol
Luciana Buriol was a professor in the Computer Science Department of the Federal University of Rio Grande do Sul (UFRGS), Porto Alegre, Brazil, for fifteen years. Since May 2021 she is a Principal Research Scientist at Amazon, Seattle/USA. Her background is in Computer Science with Ph.D. in the optimization area (State University of Campinas, Brazil, 2003). She was a visitor Ph.D. student for fifteen months at AT&T Labs (USA, 2001-2002), and a postdoc in the Computer Science Department at the University of Rome “La Sapienza” (Italy, 2004-2005). She was President of the Latin-Ibero-American Association of Operational Research (2012-2014) and Vice-President of IFORS – International Federation of Operational Research Societies (2016-2018).
Monday
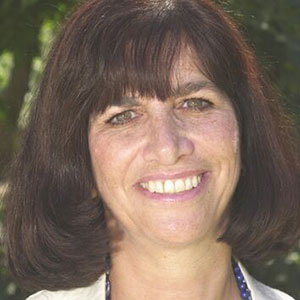
Karla Hoffman
From the Battlefield to the Gig Economy: How Hybrid Optimization can Guide Decision Making in Highly Dynamic and Unpredictable Settings
This talk describes the use of optimization to assist in real-time decision-making where solutions must be available almost instantaneously. We highlight the success of these methods in two very different settings: (a) the routing and scheduling of deliveries in gig-economy applications; and (b) the problem of sustaining communications in a highly dynamic battlefield environment. In the first of these applications, we present the problem of assigning drivers to service requests and presenting the drivers with an efficient routing of all request locations. The decision framework for these assignment problems often has competing objectives (e.g., minimizing cost to the company, providing assignments that are profitable to the drivers, and assuring that customers receive their orders in a timely fashion). The process may include predicting whether a driver will accept a given assignment and the notification to both the customer and the supplier (e.g., the restaurant providing the food to be delivered). If the drivers are not hired on a schedule, then the driver might refuse an offer and It might, therefore, take multiple “offers’ to alternative drivers before the assignment is finalized. The entire process of allocating of assignments to drivers, notifying customers of expected delivery time and alerting suppliers to new demands must be done in under a minute. In our second application, there is the need to reassign wireless channels to a military unit (or units) that lose communication capabilities due to enemy jamming or other interference issues. The need to recover quickly while assuring that reassignments do not harm other units is essential to overall battlefield success. Our approach uses hybrid algorithms designed with problem structure in mind to help satisfy challenging time requirements. In both applications, we work to generate multiple feasible solutions quickly, update prior solutions with new information, and bound the solution space. This hybrid approach obtains near-optimal solutions within the tight timeframe that these applications demand. These fast hybrid algorithms use standard optimization solvers in conjunction with feasibility checkers, constraint programming, and/or decision diagrams. These techniques are likely to be applicable in many other settings.
About Karla Hoffman
Karla Hoffman is a Professor in the Systems Engineering and Operations Research Department at George Mason University (GMU) where she served as Chair for five years. She received her D.Sc. from George Washington University in operations research. Prior to her position at GMU, she worked as a mathematician in the Center for Applied Mathematics of the National Institute of Standards and Technology (NIST) where, in1984, she was awarded the Applied Research Award. Her other awards include George Mason University’s Distinguished Faculty Award, the INFORMS Fellows Award, the INFORMS George E. Kimball Medal and the INFORMS Edelman Prize. She served as President of INFORMS in 1999 and has also served on the Executive Committees of ORSA, IFORS, and the Mathematical Programming Society. Dr. Hoffman’s primary area of research is auction design and testing, and combinatorial optimization. Her research focuses on the development of new algorithms for solving complex problems arising in industry and government. She serves as a consultant to the FCC on spectrum auctions and has previously consulted to a variety of government agencies. Her industrial consulting has been in dynamic and real-time routing and scheduling, and in capital budgeting.
Dimitris Bertsimas
Omega Rho Keynote: Artificial Intelligence and the Future of Universities
Artificial intelligence (AI) leveraging multiple data sources and input modalities (tabular data, computer vision, and natural language) is poised to become a viable method to deliver more accurate results and deployable pipelines across various applications. This work proposes and evaluates a unified Holistic AI in Medicine (HAIM), Agriculture, Meteorology, and Law. We show that our proposed framework can consistently and robustly produce models that outperform similar single-source approaches across all these applications. We also quantify the contribution of each modality and data source using Shapley values, demonstrating the importance of heterogeneity in data type and the necessity of multimodal inputs across different fields. Our Holistic AI framework’s generalizable properties and flexibility could offer a promising pathway for future multimodal predictive systems in various applications.
Throughout their entire history, universities have been organized along distinct departments. I outline what I expect will be the impact of these developments on how universities will be organized in the following decades and how OR/MS can play a leading role.
About Dimitris Bertsimas
Dimitris Bertsimas is the Boeing Professor of Operations Research and the Associate Dean of Business Analytics at the Sloan School of Management at the Massachusetts Institute of Technology. He is a member of the US National Academy of Engineering, an INFORMS fellow, recipient of the John von Neumann Theory Prize, the Frederick W. Lanchester Prize, the Erlang Prize, finalist of the Franz Edelman Prize four times, and the INFORMS President’s Award, among many other research and teaching awards, supervisor of 88 completed and 25 current doctoral theses, co-author of seven graduate level textbooks, 300+ publications, editor of the INFORMS Journal on Optimization and co-founder of ten analytics companies and two nonprofit foundations.
Jane Snowdon
The Era of Accelerated Discovery in Healthcare and Life Sciences
Technology has tremendous potential to be a primary catalyst for transformation in the healthcare and life sciences industry. Today a convergence of computing revolutions is taking place that will help accelerate the rate of scientific discovery. The era of accelerated discovery is fueled by significant advances across artificial intelligence (AI), hybrid cloud, and quantum computing combined with massive amounts of data from heterogeneous sources. In this talk, I present an overview of these information technology trends and some results obtained from applying AI and advanced analytics to provide insights for population health and precision medicine.
About Jane Snowdon
Dr. Jane L. Snowdon is an Innovation Engagement Leader and member of the venture capital and innovation team within IBM Corporate Technical Strategy. She develops the pipeline, evaluates, and leads proof-of-concepts to create strategic business value and accelerate innovation in collaboration with customers and partners.
Previously, Dr. Snowdon served as Deputy Chief Science Officer, Science Operations, IBM Watson Health, and was responsible for assisting the Chief Science Officer in developing and executing scientific strategy, integrating scientific expertise and evaluation into the product design and development process, engaging clients and academic partners to develop and execute scientific collaborations to support IBM business goals, and participating in scientific and academic forums.
Dr. Snowdon is an IBM Industry Academy Member, Fellow of the American Medical Informatics Association (FAMIA), and 2019 American Public Health Association (APHA) Achievement in Industry Awardee. She received two Watson Health General Manager’s 2021 Team Awards for “Analysis of Variants in SARS-CoV-2” and “Resetting the Clinical Trial Clock in a Pandemic-Identifying Opportunities to Accelerate the Clinical Trial Timeline to Facilitate Rapid Discovery of COVID-19 Drugs and Vaccines,” 2020 Health Tech Hero Award from the Women of IBM Watson Health, and 2020 YWCA Greenwich Women Who Inspire Award.
Dr. Snowdon served as Chief Innovation Officer, IBM Federal and Director of the IBM Federal Cloud Innovation Center in Washington DC. She conducted research for two decades at the IBM T. J. Watson Research Center in modeling, simulating, and optimizing manufacturing systems, airline crew scheduling and flight planning systems, and energy efficient buildings. She jointly led the direction of IBM’s overall Research strategy across twelve global labs and the Global Technology Outlook which became IBM’s cloud, analytics, mobile, social and security (CAMSS) strategy.
Dr. Snowdon has a proven record of successfully consulting with CxOs to drive enterprise transformation and building ecosystems with IBM’s clients, universities, business partners, and governments to enable multi-disciplinary collaboration in the development and deployment of new methods, processes, tools, and services.
Dr. Snowdon received her Ph.D. from the Georgia Institute of Technology, M.S. from the University of Michigan, and B.S. with honors from the Pennsylvania State University, all in Industrial Engineering. She has published more than 100 articles and hold 1 patent. She is a Senior Member of IIE, IEEE and SWE, and a Member of AMIA, APHA, CASE, INFORMS, NYAS, Sigma Xi, and SWE.
Maria Vlasiou
UPS Smith Winner Reprise: Learning by Doing at the Eindhoven University of Technology
The Eindhoven University of Technology (TU/e) was founded in 1956 in the spirit of collaboration with society and industry. This spirit is still at the heart of the university community. TU/e has ranked consistently as one of the top performing universities worldwide in collaboration and scientific output in cooperation with industry. The Industrial and Applied Mathematics (IAM) Master’s program of TU/e embodies this principle. The program offers academic education that is driven by fundamental and applied research in close collaboration with industry. Our educational philosophy is based on personal attention and room for individual ambitions and talents and supports a fully individualized study program. Our program combines scientific curiosity with a hands-on mentality. Fundamental knowledge enables us to design solutions for the highly complex problems of today and tomorrow. We understand things by making them and we make things by understanding them. For more than 50 years, the Mathematics and Computer Science Department has partnered with industry, produced impactful research, and influenced the teaching of operations research. In this presentation, we provide an overview of the historical and current innovative activities undertaken by IAM that culminated in its receiving the 2022 INFORMS UPS George D. Smith Prize.
About Maria Vlasiou
Maria Vlasiou is a professor in the Electrical Engineering, Mathematics, and Computer Science Department at the University of Twente, The Netherlands, a Research Fellow of the European research institute EURANDOM, and an associate professor in the Mathematics and Computer Science Department at the Eindhoven University of Technology (TU/e), where she serves as associate Chair of the stochastics cluster. In addition, she represents the Netherlands at the European Women in Mathematics network and the Committee for Women in Mathematics of the International Mathematical Union, and is a board member of the Dutch women in mathematics association, where she has served as a Chair for five years. Dr. Vlasiou’s primary area of research is on the performance of stochastic processes. Her research focuses on processes acting on interacting networks, developing performance estimates and control mechanisms for complex problems arising in high-tech manufacturing, energy networks, and mathematical biology. Her research has received the ICORES 2013 Best Paper Award, the Marcel Neuts Student Paper Award, and the 3rd prize in the 8th conference in Actuarial Science.
Tuesday
Brian MacDonald
Sports Analytics
Data has been meticulously collected in sports for decades. As technology has improved, so has the data, allowing for more appropriate statistical modeling and machine learning techniques to be used to answer interesting questions. Today, many leagues use computer vision, remote sensing, and machine learning techniques to collect player and ball location data multiple times per second throughout the duration of every game, and to automatically detect events of interest during a game, both of which allow for numerous new types of analyses. We will give a brief history of analytics in sports, the questions that teams, leagues, media organizations and fans try to answer using data, the current state of analytics in the sports industry, and how sports analytics is being used in education to inspire student interest in data science.
About Brian MacDonald
Jonathan Eckstein
Parallel Computing and OR/MS
Parallel computing is currently a widespread tool in science and engineering. It is now common for large universities, large corporations, and government labs to use HPC (high-performance computing) systems with tens of thousands of processor cores for applications such as protein folding prediction, other kinds molecular simulations, fluid flow simulation, performance analysis of physical structures, fossil fuel reservoir analysis, weather prediction, and neural network training. By comparison, operations research as a whole makes minimal use of large-scale parallel computing technology. I will discuss why this is the case, and the potential for making better use of the technology in our field.
I will describe the basics of parallel computing, and how the available hardware and software has evolved since the 1980s. Arguably, parallel programming tools have not matched the pace of hardware evolution and do not provide an ideal level of abstraction for software development. This situation has lead to uneven use of technology between various fields, depending on the availability of monolithic task-specific software applications and libraries. In operations research, a key obstacle is the challenge of adapting commonly used algorithms for continuous and mixed-integer optimization to the presently available parallel hardware and coding environments.
However, the potential for using parallel computing in operations research remains, and the benefits could be substantial. In particular, I will examine how numerical optimization solvers might more effectively take advantage of parallel computing, considering both altered approaches to continuous optimization and better parallel implementation of search methods for discrete and non-convex optimization. Promising applications include difficult mixed-integer programming problems, control of large-scale power grids under uncertainty in both supply and demand, and mixed continuous-discrete data fitting problems.
About Jonathan Eckstein
Jonathan Eckstein is a Distinguished Professor in the department of Management Science and Information Systems at Rutgers University. His principle research interests are in numerical optimization algorithms, both continuous and discrete, and especially their implementation on parallel computing platforms. Areas of particular focus include augmented Lagrangian/proximal methods, branch-and-bound algorithms, and stochastic programming. He has also worked on risk-averse optimization modeling and on applying O.R. techniques to managing information systems. He completed his Ph.D. in operations research at M.I.T. in 1989, and then taught at Harvard Business School for two years. He then spent four years in the Mathematical Sciences Research Group of Thinking Machines, Inc. before joining Rutgers. At Rutgers, he led an effort establishing a new undergraduate major in Business Analytics and Information Technology (BAIT). In 2014, he was elected a fellow of INFORMS.In 2019, he became editor-in-chief of the journal Mathematical Programming Computation.
Peter Frazier
Modeling for COVID-19 College Reopening Decisions: Cornell, A Case Study
Like all universities, in the summer of 2020 Cornell faced the question of whether to go online-only in the fall or to bring students back for a residential semester. Like many universities, Cornell decided on a residential semester. Like few universities who invited all students back, Cornell managed to keep COVID-19 at bay for the entire semester. Our modeling team played a major role in the decision to reopen, perhaps paradoxically showing that with appropriate interventions a residential semester was safer than an online-only one. Our team also helped design the major interventions deployed, including asymptomatic surveillance through pooled testing on-site, adaptive testing and a complete rebuild of the class schedule. The arrival of the Alpha variant in the spring of ’21 and the Delta variant in fall of 2021 caused us to repeatedly strengthen our interventions, again only after modeling showed the potential benefits. The explosive arrival on campus of Omicron late in the fall 2021 semester led to a major change of strategy, again guided by the work of the modeling team. All of this work required great care in capturing uncertainty in key input parameters such as asymptomatic infection rates and, more recently, vaccine effectiveness. We’ll share a perspective on this wild ride, emphasizing our modeling approaches, our close collaboration with Cornell leadership and some funny (in retrospect) stories.
About Peter Frazier
Peter Frazier received a B.S. in physics and engineering/applied science from the California Institute of Technology in 2000, after which he spent several years in industry as a software engineer, working for two different start-up companies and for the Teradata division of NCR. In 2005, he entered graduate school in the Department of Operations Research & Financial Engineering at Princeton University, and received an M.A. in 2007 and a Ph.D. in 2009. He joined the faculty at Cornell in 2009 as an Assistant Professor in the School of Operations Research & Information Engineering, where he is now an Associate Professor. His research is in sequential decision-making under uncertainty, optimal methods for collecting information, and machine learning, focusing on applications in simulation, e-commerce, medicine, and biology. He is the recipient of a CAREER Award from the National Science Foundation and a Young Investigator Award from the Air Force Office of Scientific Research. He is currently on leave at Uber, where he is a Staff Data Scientist and Data Science Manager. At Uber, he worked on UberPOOL from 2015-17, and on broader pricing efforts from 2016-17. He now leads a data science team focused on pricing.
Shane Henderson
About Shane Henderson
Shane G. Henderson is the Charles W. Lake, Jr. Professor in Productivity in the School of Operations Research and Information Engineering at Cornell University. His overall professional goal is to contribute to both research and learning in the theory and application of stochastic simulation and applied probability, with emphasis on the interface between these areas and optimization. He is greatly interested in and motivated by applications with strong societal relevance, including bike sharing, medical scheduling, and ambulance planning.
David Shmoys
About David Shmoys
David B. Shmoys is the Laibe/Acheson Professor and Director of the Center for Data Science for Enterprise & Society at Cornell University. He obtained his Ph.D. in computer science from the University of California at Berkeley in 1984, and held postdoctoral positions at MSRI in Berkeley and Harvard University, and a faculty position at MIT before joining the faculty at Cornell University. He was Chair of the Cornell Provost’s “Radical Collaborations” Task Force on Data Science and was co-Chair of the Academic Planning Committee for Cornell Tech. His research has focused on the design and analysis of efficient algorithms for discrete optimization problems, with applications including scheduling, inventory theory, computational biology, computational sustainability, and most recently, data-driven decision-making in the sharing economy. His work has highlighted the central role that linear programming plays in the design of approximation algorithms for NP-hard problems. His book (co-authored with David Williamson), The Design of Approximation Algorithms, was awarded the 2013 INFORMS Lanchester Prize and his work on bike-sharing (joint with Daniel Freund, Shane Henderson, and Eoin O’Mahony) was awarded the 2018 INFORMS Wagner Prize. David is a Fellow of the ACM, INFORMS, and SIAM, and was an NSF Presidential Young Investigator.
2022 Daniel H. Wagner Prize Winner Reprise
The Daniel H. Wagner Prize is awarded for a paper and presentation that describe a real-world, successful application of operations research or advanced analytics. The prize criteria emphasizes innovative, elegant mathematical modeling and clear exposition. To learn more about the prize, visit the information page.