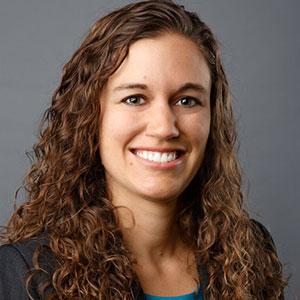
Kris Ferreira
Kris Ferreira
Assistant Professor, Technology and Operations Management Unit at Harvard Business School
Kris Ferreira is an Assistant Professor at Harvard Business School in the Technology and Operations Management (TOM) Unit. She teaches analytics in a variety of Executive Education programs. In her research, Kris focuses on helping e-commerce companies make more profitable revenue-management decisions, including pricing, assortment planning, ranking, etc. For much of this work, she partners with online retailers to design new machine learning and optimization algorithms that use the massive amount of data at their fingertips to improve their decision making in this domain. Most recently, Kris has started studying how users make decisions with such algorithmic input, and correspondingly how to improve algorithmic development and deployment. Kris’s work has been awarded or named a finalist for the M&SOM Best Paper Award (two-time), M&SOM Best Operations Management Paper in Operations Research Award, M&SOM Practice-Based Research Award, INFORMS Revenue Management & Pricing Section Practice Award (two-time), and INFORMS Innovative Applications in Analytics Award. Kris earned her PhD in Operations Research at the Massachusetts Institute of Technology. Prior to her PhD, Kris worked as a consultant for Alvarez & Marsal.
Associate
Professional
Track: Revenue Management & Pricing
Data-Driven Pricing
For the first part of the session, I will describe how to apply and combine descriptive, predictive, and prescriptive analytics to identify opportunities, predict demand, and make pricing decisions. Much of this will focus on the predictive analytics piece, where I will explain what machine learning is and how it can be used in demand predictions which feed into pricing decisions. Throughout this part, I will share several examples from my research where I have partnered with companies to develop and implement machine learning and optimization algorithms for demand prediction and pricing. A big challenge that many companies face when implementing analytics as decision support tools – providing recommendations as inputs for human users when making their final decisions – is that users oftentimes make errors when using algorithms, discounting or adhering to algorithmic recommendations when they shouldn’t be. These errors are incredibly costly: simply put, even if an organization has access to outstanding algorithms, if users do not effectively incorporate them in their decision making, the organization will not realize the algorithms’ full potential. In the second part of the session, I will present new research that starts to uncover why and when users make errors, and I will share recommendations regarding how to improve their use of algorithms. I am actively looking for industry partners to collaborate and further explore this line of research.