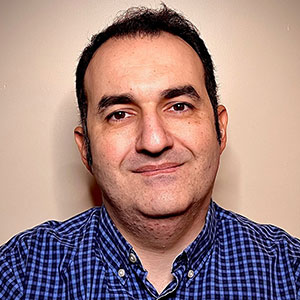
Ulas Cakmak
Ulas Cakmak
Senior Director, Optimization Science at Infor
Ulas Cakmak is a Senior Director in the Optimization and Analytics team at Infor. For over 10 years, Ulas participated in both product development and implementation efforts with a focus on building cutting-edge algorithms and delivering provable practical value to Infor clients. His work centered around employing machine learning and optimization techniques to solve complex supply chain problems and covered various fields, including forecasting, demand planning, replenishment, markdown and price optimization. Prior to Infor, Ulas worked as a supply chain consultant at Agility Logistics for three years. Ulas holds a Ph.D. in Industrial Engineering from Georgia Institute of Technology.
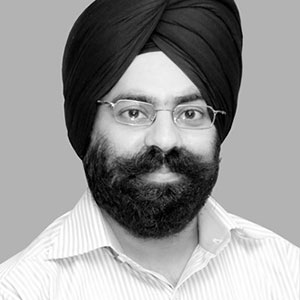
Inderjeet Singh
Inderjeet Singh
Senior Director, Decision Science at Infor
Inderjeet Singh is a Senior Director in the Applied Innovation group at Infor. He manages the Decision Science COE responsible for achieving measurable and repeatable value for enterprise customers from their investments in Infor Machine Learning. Inderjeet has 12+ years of industry experience in operationalizing large-scale predictive and prescriptive analytics to solve business problems in demand forecasting, predictive maintenance, and operational process control across retail, energy, manufacturing, process, and service industries. Before Infor, Inderjeet worked as a Scientist at the Indian Space Research Organization. He holds a Ph.D. in Operations Research and Industrial Engineering from the University of Texas at Austin.
Associate
Professional
Track: Revenue Management & Pricing
Adapting Forecasting Algorithms to Accommodate Disruptions
In many industries supply chain disruptions have always been a part of business and recent tumultuous times emphasized the importance of the right way of handling them. Frequently occurring minor disruptions are often ignored in operational planning, likely because these are not expected to cause serious issues as long as they are not widespread. Most modern Machine Learning methods can indeed mitigate the risk caused by randomly spread minor data anomalies. However, even the best forecasting models are susceptible to invalid data points caused by large and persistent supply chain disruptions. We present a forecasting approach combining Machine Learning and traditional timeseries based techniques. This flexible approach ornamented with outlier detection and lost sales estimation allows us to build forecasting systems that are robust against supply chain disruptions. Machine Learning provides the ability to estimate the impact of various causal factors from a holistic viewpoint, while traditional methods ensure the lower-level characteristics are not lost in the process. Moreover, these systems provide critical input needed in making pricing, promotional and markdown decisions.