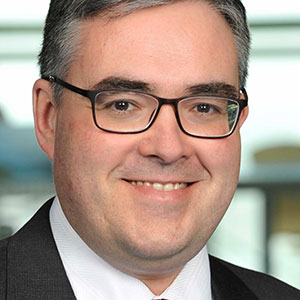
Miguel Anjos
Miguel Anjos
Chair of Operational Research at the School of Mathematics, University of Edinburgh
Miguel F. Anjos holds the Chair of Operational Research at the School of Mathematics, University of Edinburgh. He was previously on the faculty of Polytechnique Montreal, the University of Waterloo, and the University of Southampton. His accolades include a Canada Research Chair, the NSERC-Hydro-Quebec-Schneider Electric Industrial Research Chair, a Humboldt Research Fellowship, the title of EUROPT Fellow, and the Queen Elizabeth II Diamond Jubilee Medal. He is a Fellow of the Canadian Academy of Engineering.
Miguel’s research interests are in the theory, algorithms, and applications of mathematical optimization. He has had extended research collaborations with companies such as EDF, Expretio Technologies, Hydro-Quebec, National Grid, Rio Tinto, and Schneider Electric. He is the Founding Academic Director of the Trottier Institute for Energy at Polytechnique Montreal. He has been an active INFORMS member for nearly 20 years and served on the Advisory Council for the INFORMS Practice Conference from 2007 to 2011, and on the Selects Committee for the INFORMS Business Analytics Conference from 2013 to 2017. Currently he is the INFORMS VP for International Activities, and President of the INFORMS Section on Energy, Natural Resources, and the Environment.
Professional
Track: Forecasting
An Inexpensive Machine Learning Approach for Robust Forecasting, Or How to Fix the Forecasting Models that the Pandemic Broke
The Covid-19 pandemic’s effect on consumer behaviour caused many predictive models to completely fail. As this predictive work is essential in downstream decision making, especially in such a time of crisis, a reliable and efficient method to mend the predictive capacity of forecasting models is a crucial business need. This presentation is about a method developed at ExPretio Technologies to tackle this problem for rail passenger demand forecasting. The key insight is that existing models can be recycled as inputs for machine learning, specifically to reassess the predictions from the original models in the context of newly incoming data. A major advantage of this method is that it alleviates the need for costly calibration required to develop predictive models from scratch. This method not only fixed the models broken by the pandemic but also gives an improved performance compared to previously used individual models on pre-pandemic data.