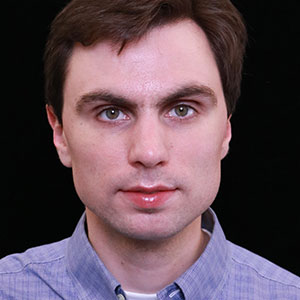
Will Bishop
Will Bishop
Data Scientist at Amazon
Will Bishop has been a data scientist at Amazon since 2019, working in the Buying team within Supply Chain Optimization Technologies (SCOT). His work there has focused on identifying anomalies within Amazon’s automated buying system, researching ways to improve the buying system’s assumptions about customer demand, and supporting other teams’ use cases for automated buying data. Before joining Amazon, he completed a M.S. in Data Science at the University of Washington in 2019, and previously worked in tax revenue forecasting for the state of Alaska.
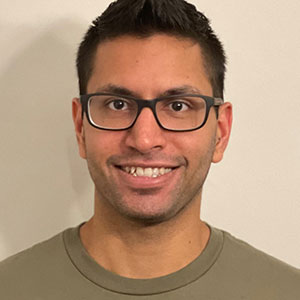
Pratik Mital
Pratik Mital
Research Science Manager at Amazon
Dr. Mital completed his Ph.D. in Industrial Engineering from Georgia Institute of Technology in 2015. After his Ph.D., he worked at Revenue Analytics for more than 5.5 years where he led the science team within the travel and transportation vertical. After that, he joined Amazon where he has been working on projects related to anomaly detection, buying schedule optimization, sub-same day fulfillment, and direct import buying. He is currently leading the data science team within the North America Fulfillment Network team. He has numerous publications in the application of advanced analytics across domains such as revenue management, supply chains, material handling systems, and education systems.
Associate
Professional
Track: Revenue Management & Pricing
Anomaly Detection in Automated Buying Systems
Automated buying systems at Amazon order hundreds of billions of dollars worth of inventory each year into thousands of fulfillment centers across dozens of countries and millions of products. These systems provide hundreds of billions of vendor-product inbound recommendations each year. These decisions are made at the last responsible moment to maximize long term free cash flow and provide an optimal service level to the customers. These models use inputs from 100+ systems and consider factors such as demand, product price, vendor cost, vendor lead time uncertainty, vendor constraints, supply risk, variability of economic terms, customer demand conversion, salvage value, and the value of being in stock at various delivery speeds while adhering to network constraints. This necessitates development of automated systems to identify defective inputs which would have resulted in sub-optimal decisions. In this talk, we present machine learning based automated anomaly detection approaches to mitigate impact of defective inputs by building awareness of historical context into real-time buying decisions. The goal of these approaches is to stop egregious automated buys and reduce reaction time to large scale changes in inputs/outputs. This has a direct impact on Amazon’s profitability. We will also share real-world examples as part of this talk.