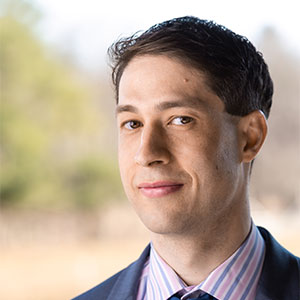
David Hampton
David Hampton
Senior Data Engineer at DCI Solutions
Executive
Track: Cyber Analytics
CyFormer: Transforming Security Through Real Time Explainable Analytics
CyFormer harnesses the power of Artificial Intelligence (AI) for the purpose of transforming cybersecurity. This collection of custom built, Natural Language Processing (NLP) models can defend against never-before-seen cyber attacks at both the network and host level. CyFormer covers all aspects of the cyber threat, both from inside and outside adversaries, harnessing the power of GPUs to scale from edge to enterprise systems. Unlike signature based solutions, CyFormer does not to rely on known threats of the past, allowing it to track down variations to past threats and stop zero day attacks in their tracks. CyFormer applies seamlessly as part of any network architecture and is a flawless technology insertion into a Zero-Trust Architecture.
CyFormer uses light-weight, custom-built Transformer models, specifically designed to address the unique challenges of cyber data. Internal representations are developed of each network entity and their relationships, thus allowing real-time, scalable alerting when unusual events happen. CyFormer can act as a stand-alone solution or can be used to augment security professionals by spotting hard-to-see anomalies in the data and explaining what makes the activity suspect. This methodology helps to build deep trust while augmenting a security team to effectively investigate the vast scale of cyber data in a meaningful, systematic, and straightforward way.