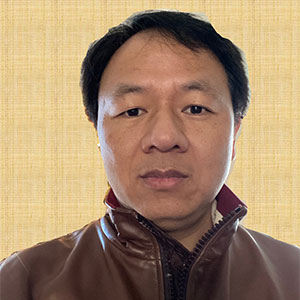
Heping Liu
Heping Liu
Senior Machine Learning Principal at Workday, Inc.
Track: Platforms & SaaS
Demand Planning of Infrastructure Resources Based on AutoML Time Series Forecasting and Assignment Optimization Model
This talk presents a demand planning framework of infrastructure resources in IT companies. The framework is based on AutoML time series forecasting and assignment optimization model. AutoML time series forecasting is developed under the architecture of big data and distributed computation, and it has a highly generalized forecasting engine powered by AutoML and big data ETL (extract, transform, load). It has the linear scalability and can enable the automatically fitting and forecasting of millions of time series forecasting models. With the forecasting output of resources, the assignment optimization model can determine how to assign infrastructure resources to individual tenants in order to minimize the usage of total infrastructure resources in an IT company for any future time within the forecasting horizon.