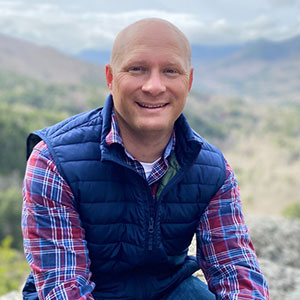
Nathaniel Bastian
Nathaniel D. Bastian
Chief Data Scientist and Senior Research Scientist at Army Cyber Institute
As a Lieutenant Colonel in the U.S. Army serving as Academy Professor and Cyber Warfare officer at the United States Military Academy (USMA) at West Point, Nathaniel D. Bastian, PhD is Chief Data Scientist and Senior Research Scientist at the Army Cyber Institute (ACI), as well as Assistant Professor of Operations Research and Data Science with a dual faculty appointment in the Department of Systems Engineering and the Department of Mathematical Sciences. Nate leads the ACI’s Data and Decision Sciences Division while overseeing the ACI’s infrastructure, platform, tooling and pipelines for data engineering and management, advanced analytics, algorithm and model development, modeling and simulation, and testing and evaluation. He directs the ACI’s Intelligent Cyber-Systems and Analytics Research Lab and the ACI’s Internet of Things Research Lab, as well as co-directs the ACI’s Cyber Modeling and Simulation Research Lab. As Principal Investigator and Technical Program Manager, his basic and applied research and development portfolio, in collaboration with Government, Academia, Industry, FFRDC, UARC and National Lab partners, aims to advance the body of knowledge in operations research, data science, artificial intelligence, machine learning, statistics, scientific computing, applied mathematics, and cognitive science to support the cyber and information domain for stakeholders across the Army, other Services, Department of Defense (DoD), Intelligence Community (IC), and Nation. Nate has co-authored over 80 refereed journal and conference papers, six book chapters, and one textbook. He is the recipient of numerous academic awards and honors, to include a Fulbright Scholarship and National Science Foundation Graduate Research Fellowship, and he has received $4M+ in externally-funded research monies from multiple government organizations. His prior military assignments include serving as Chief Artificial Intelligence Architect at the DoD Joint Artificial Intelligence Center, Operations Research Scientist at the ACI/USMA, Analytics Officer at the U.S. Army Human Resources Command, and Aeromedical Evacuation Officer and UH-60 Black Hawk Aviator at the 25th Combat Aviation Brigade. Nate earned his Ph.D. in Industrial Engineering and Operations Research from the Pennsylvania State University (PSU), M.Eng. in Industrial Engineering from PSU, M.S. in Econometrics and Operations Research from Maastricht University, and B.S. in Engineering Management (Electrical Engineering) with Honors from USMA. He is an active professional member of INFORMS, MORS, ACM, IEEE, and AAAI.
Executive
Track: Cyber Analytics
Data Augmentation to Improve Adversarial Robustness of AI-Based Network Security Monitoring
Cyber security is an international challenge that is increasingly important as the inter-connectedness of the world grows. The reliance of systems on computational assets makes them vulnerable to attack. Traditionally, networks and systems are monitored by cyber security operators who rely on intrusion detection systems to provide indicators of compromise via alerts. With the growing number and frequency of alerts and the increasing sophistication of attacks, human operators are incapable of keeping pace. Data-driven and statistical tools, such as algorithms from artificial intelligence (AI), have the potential to assist in this area. These AI technologies enable the collection of synchronized, real-time capabilities to discover, define, analyze, and mitigate cyber threats and vulnerabilities with limited human intervention. Network intrusion detection systems (NIDS) are a primary component of the broader practices in network security monitoring for cyber security operations at enterprise, operational and tactical environments. Today, integrating AI components into NIDS can help identify patterns associated with known threats or detect abnormal behavior. These AI-based NIDS, however, are susceptible to adversarial AI evasion attacks. In this talk, I will discuss how data augmentation techniques can be used to improve the adversarial robustness of AI-based NIDS for network security monitoring. Particularly, I will discuss how meta-heuristics can be used to generate adversarial examples to then be combined as part of a meta-learning adversarial training framework.