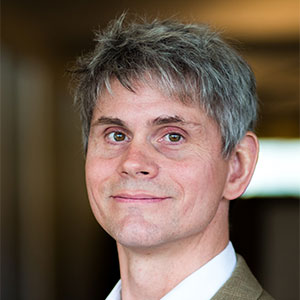
Scott Zoldi
Scott Zoldi
Chief Analytics Officer at FICO
Scott Zoldi is chief analytics officer at FICO responsible for advancing the company’s leadership in artificial intelligence (AI) and analytics in its product and technology solutions. At FICO Scott has authored more than 120 analytic patents, with 80 granted and 47 pending. Scott is actively involved in the development of analytics applications, Responsible AI technologies and AI governance frameworks, the latter including FICO’s blockchain-based model development governance methodology – and was most recently awarded the Future Thinking Award at Corinium’s Business of Data Awards Gala. Scott is a member of the Board of Advisors of FinRegLab, a Cybersecurity Advisory Board Member of the California Technology Council, and a Board Member of Tech San Diego and the San Diego Cyber Center of Excellence. Scott received his Ph.D. in theoretical and computational physics from Duke University.
Track: Behavioral Analytics
Responsible AI: Maturity in AI Development
This paper presents an empirical analysis of health insurance claims data to explore telemedicine outcomes. Specifically, I utilize causal forests and a retrospective matched case control study design to demonstrate statistically significant changes in costs, utilization, and medication adherence of telehealth users. Every other day brings news of how AI has misbehaved, concerns of unethical or unsafe use, and complexity outweighing data scientist understanding. While AI provides a great business opportunity, increasing social awareness and regulation point to the need for thoughtful, demonstrated proper development of models and AI systems. Regulators have stepped in with a slew of regulatory frameworks such as GDPR, AI Act and IEEE standards to help current and future standards to regulate AI. Increasingly mature organizations are focusing on AI and machine learning built at the same level of sophistication and with defined/monitored processes as software. The first step in that journey requires firm model development governance standards across the corporation that ensure alignment to these corporate standards and demonstrated accountability to following the standard during model development. This contrasts with the older methods of inferring rightness/wrongness of models built afterwards by model governance teams. Interpretability of the model is key to achieving transparency and explainability. We will review novel interpretable latent feature based neural networks that allow for the required transparency in responsible AI applications. This transparency enables explainability of the model through model architecture (as compared to inference methods), for example, by using highlander constraints to limit each hidden node connections. This forces the algorithm to find a solution which is inherently interpretable, where each hidden node can be easily explained in terms of non-linear interaction terms that are easy to understand, test, and defend by human experts. This transparent architecture also enables bias testing of the latent features across protected classes. This makes it possible to drive ethics testing through exposing learnt latent features (which drive model outcomes) for bias. Once offending imputed relationships are exposed, they can be eliminated from the model in a retrain process. Further, when the model is deemed ethical specific thresholds for bias drift are also produced for monitoring.Auditability is accomplished through configuration of a model development governance blockchain that codifies and enforces the corporation’s model development standards. An auditable and persistent record of all actions and decisions pertaining to the model and related asset development as well as production of quantities for continuous monitoring in production is maintained on blockchain. The combination of enforcing model development standards and monitoring that are maintained in production through Auditable AI is key to responsible AI. Together these three tenants of responsible AI drive the standards, processes, and monitoring required to ensure that responsible AI development can mirror software development processes/tools already in place for decades for critical software applications.