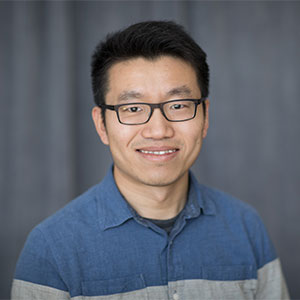
Zhiwei (Tony) Qin
Zhiwei (Tony) Qin
Principal Scientist at Lyft Rideshares Lab
Tony Qin is Principal Scientist at Lyft Rideshare Labs, working on core problems in ridesharing marketplace optimization. Previously, he was Principal Research Scientist and Director of the Decision Intelligence group at DiDi AI Labs. Tony received his Ph.D. in Operations Research from Columbia University. His research interests span optimization and machine learning, with a particular focus in reinforcement learning and its applications in operational optimization, digital marketing, and smart transportation. He is Associate Editor of the ACM Journal on Autonomous Transportation Systems. He has served as Area Chair/(Senior) Program Committee of KDD, AAAI, NeurIPS, ICML, IJCAI, and a referee of top academic journals. He is an INFORMS Franz Edelman Award Finalist in 2023, received the INFORMS Daniel H. Wagner Prize for Excellence in Operations Research Practice in 2019 and was selected for the NeurIPS 2018 Best Demo Awards. Tony holds more than 10 US patents in intelligent transportation, supply chain, and recommendation systems.
Track: Travel & Hospitality
A Market State Representation Framework for the Two-sided Marketplace Of Ridesharing
Ridesharing platforms are a particular type of two-sided marketplace where `supply-demand (SD) balance’ is critical to the market efficiency and is also complex to analyze. We describe an analytical framework based on the graph equilibrium metric (GEM) for quantifying the supply-demand spatiotemporal state of a ridesharing marketplace. GEM was developed as a generalized Wasserstein distance between the supply and demand distributions in a ridesharing market and has been used as an evaluation metric for algorithms expected to improve SD alignment. We extend the GEM framework to support a dual-view (supply and demand side) of balance in a market. We show that there are often disparities between the two views and how this dual-view leads to the notion of market efficiency, in which we propose a test statistic for capturing improvement.